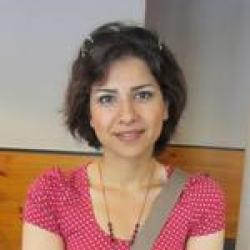
A Graphical model is a probabilistic model for which a graph is used to represent the conditional independence between random variables. Such models have become extremely popular tools for modeling complex real-world systems. Learning graphical models is of fundamental importance in machine learning and statistics and is often challenged by the fact that only a small number of samples are available relative to the number of variables. Several methods (such as Graphical Lasso) have been proposed to address this problem. However, there is a glaring lack of concrete case studies that clearly illustrate the limitations of the existing computational methods for learning graphical models. In this talk, I will propose a circuit model that can be used as a platform for testing the performance of different statistical approaches. I will show that the data generated from this circuit model exhibits similar trends to resting-state functional MRI (fMRI) data, and then discuss how our findings from case studies of the circuit model can be used to study graphical models for brain functional connectivity from fMRI data. Motivated by the above results, I will develop new insights into regularized semidefinite program (SDP) problems by working through the Graphical Lasso algorithm. Graphical Lasso is a popular method for learning the structure of a Gaussian model, which relies on solving a computationally-expensive SDP. I will derive sufficient conditions under which the solution of this large-scale SDP has a simple formula, and test them on electrical circuits and fMRI data.
Phone: 858-534-4254
Email: yhk@ucsd.edu