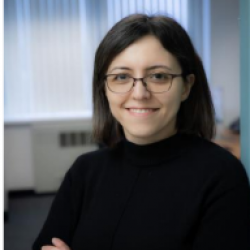
In this talk, I will focus on two challenging imaging systems: snapshot compressive imaging and coherent imaging under speckle noise interference. I will begin by reviewing the core mathematical modeling of the inverse problem corresponding to each system. I will develop a maximum likelihood estimator (MLE)-based optimization for each, employing untrained neural networks (NNs) to model the source structure. Theoretical analysis of the MLE-based methods will be shown to enable, on one hand, an understanding of the fundamental limits of these systems and, on the other hand, optimization of the image recovery algorithms and hardware. I will also discuss our proposed algorithms that merge classic bagging ideas with untrained neural networks for solving the inverse problems in these imaging systems. For each application, I will demonstrate how our method achieves state-of-the-art performance.